Humans are wired to spot patterns, from language learning to number recognition, and this ability is key to our survival. It is no surprise, then, that we have leaned on this skill to expand our knowledge and make better-informed decisions. Over time, we have developed ways of processing data to reveal useful information, such as uncovering the most urgent needs of a community through census results.
While small amounts of information can be tackled without much difficulty, large datasets require more sophisticated mechanisms, and this is where advanced analytics come in. Simply put, advanced analytics use a set of tools and computational techniques to uncover patterns that would difficult to spot with the naked eye. The advanced analytics toolbox includes machine learning, data mining, and visualizations, helping the user by providing the required data in a way that is easy yet powerful. An example of advanced analytics in action is the COVID dashboard provided by the Robert Koch Institute in Germany.
How are advanced analytics beneficial?
To truly grasp the benefits of advanced analytics, it helps to look at concrete scenarios where these techniques have made a difference, like in the following pharmaceutical companies.
Monitoring drug production
To ensure that their pharmaceutical manufacturing process produces consistent results, Novartis has started to employ advanced analytics. Instead of carrying out spot tests for specific batches at specific points of the process, they use techniques to detect globally whether there are any potential production issues. With the ability to spot these problems early, they can prevent batches from going to waste.
Suggesting personalized treatments
Moving beyond the traditional one-size-fits-all approach, Sanofi has been investing in advanced analytics techniques to understand what patients benefit the most from what type of care. By analyzing complex background information, their aim is to provide personalized recommendations to optimize people’s treatment. This improves outcomes and streamlines healthcare.
Accelerating drug discovery
To improve the success rate of new candidate drugs at trials, Evotec has developed advanced analytics-based platforms that can accelerate the identification of promising compounds and more accurately predict their efficacy. With the help of these techniques, they can make faster decisions on which candidates warrant further investigation.
What are examples of tools for advanced analytics?
The techniques used for achieving these outcomes depend on the particular needs of the company or project. The following are examples of popular tools for advanced analytics:
Clustering
Clustering algorithms separate data points into distinct classes, so that similar points are grouped under a common category. When comparing two points to evaluate whether they belong together, certain clustering algorithms use distance measures to find the best category fit. Clustering is an ideal way of understanding the natural divisions within a dataset.
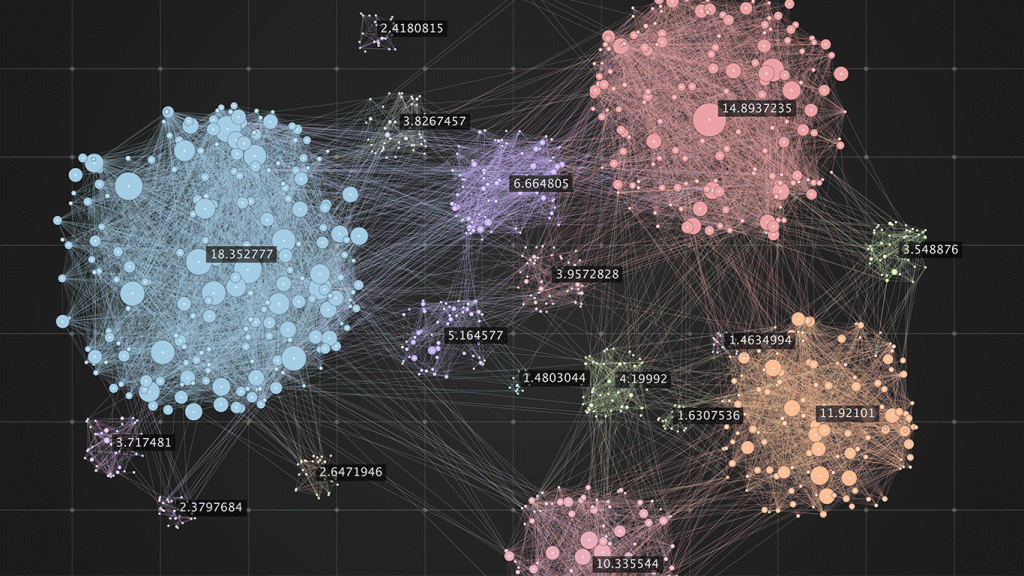
Image source: Adobe Stock, ID: 269630614
Statistical models
Another way of gleaning insights from data is by modelling it statistically, which reveals underlying assumptions and relationships between different variables. Statistical models are an ideal first step for data analysis, as they can provide intuitive results even with comparatively little available data. Some of these methods are “explainable”, meaning that one can easily understand why a method has arrived at a particular outcome, which may be a business requirement in certain environments.
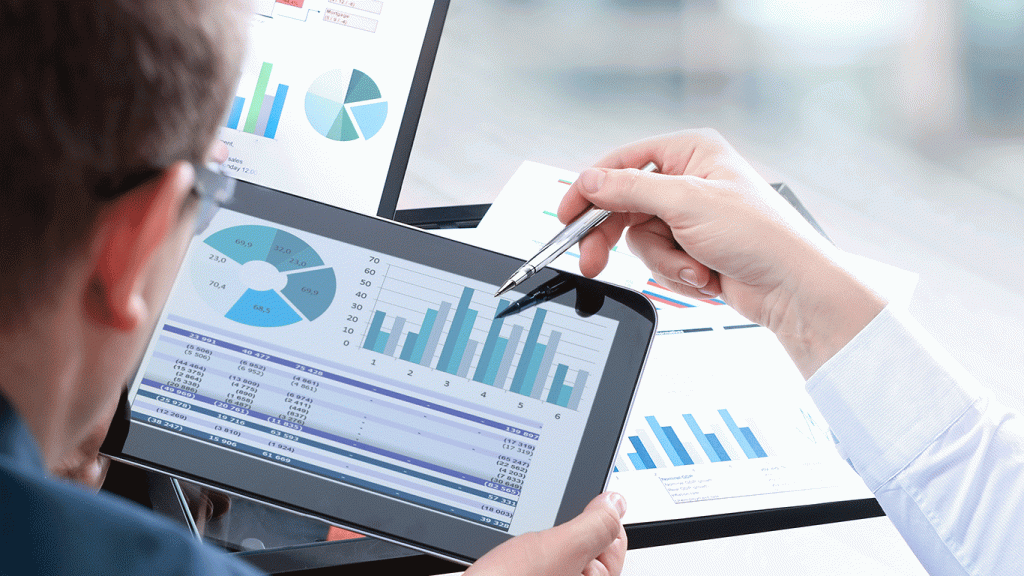
Image source: Adobe Stock, ID: 38523635
Time-Series analysis
Time-series are sequences of data of where each datum is associated with a timestamp. Many processes produce time-dependent data, ranging from stock markets over epidemiological processes to growth rates in bio-reactors. Analysis of time-series requires specialized analytical methods. A very common use-case is the detection of extraordinary events in a time-series, called anomaly detection.
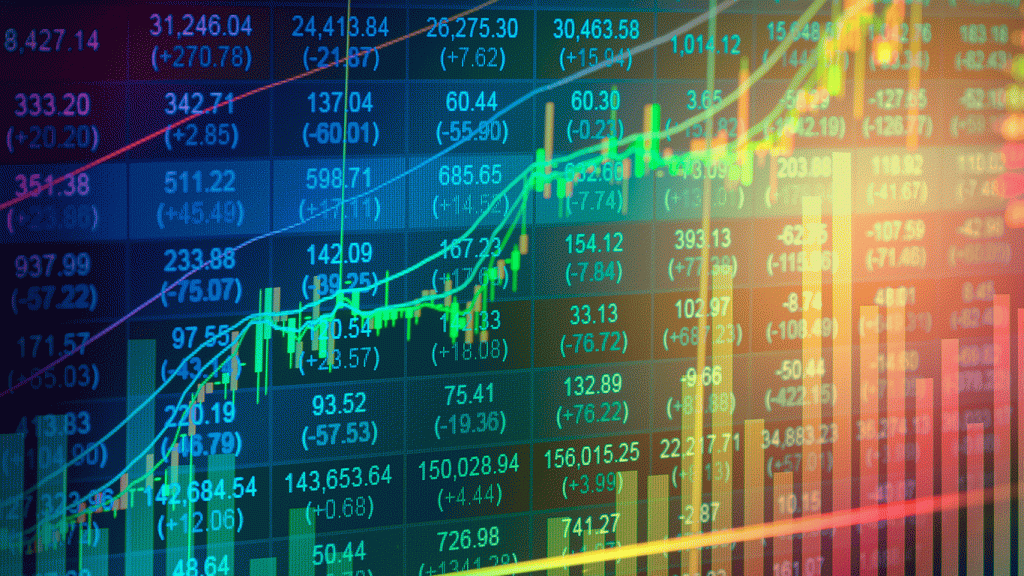
Image source: Adobe Stock, ID: 349697255
Neural networks
A neural network is a learning system that is inspired by the structure of a biological brain, with interlinked units of computation that represent neurons. Data is fed into the network and propagated through these units, allowing the system to gradually learn patterns that it can identify later. Neural networks are cutting edge and can provide very good results, but typically require a lot of effort and data to develop and maintain.
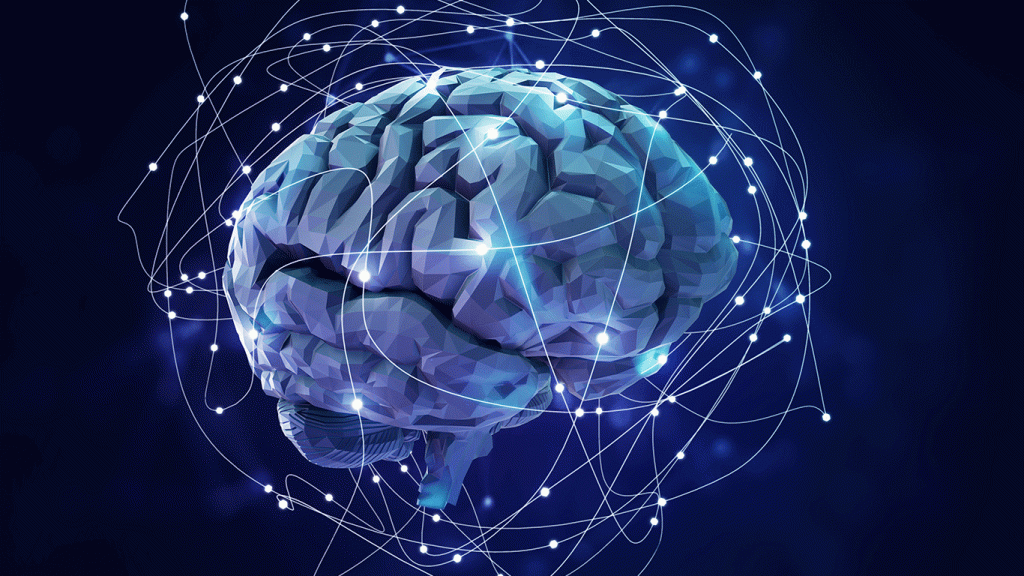
Image source: Adobe Stock, ID: 315930845
How to get started?
The following steps can get you started with data analytics:
- Set clear goals and requirements: before even configuring a system or looking at the data, you must have a clear understanding of the overall goal. For instance, is the objective to categorize data somehow? Would you like to create some form of forecast? Also consider other requirements: should the resulting analytical method be regularly updated with new data? Are there regulatory requirements for production use? Do you require an explainable result?
- Make your data FAIR: next, ensure that the data you want to leverage is easily findable, accessible, interoperable, and reusable (g., in a common and well-documented format). Ideally, all information would be centralized under a single repository, providing a standardized interface through which the data can be accessed. For many advanced analytics projects, most efforts are spent in cleaning up data.
- Proof-of-Concept (PoC): once the goals and the data infrastructure are clear, it is time to create a proof-of-concept implementation. This requires expertise and occasionally some experimentation to accomplish the stated goal. A PoC is meant to highlight the benefit of using advanced analytics in a low-cost and low-risk setting. As such, a comprehensive assessment of the results and collection of end-user feedback are crucial at this step.
- Industrialize: with all pieces of the puzzle in place, you can roll out your advanced analytics capability. Typically, the roll-out includes an operational concept, change management, and monitoring infrastructure.
If you need any support throughout your data analytics journey, do not hesitate to contact OSTHUS. Our experts will be happy to help set up the best solution for your organization. Check out our case-study where we helped improve the prediction of next years’ influenza strain using Advanced Analytics techniques.